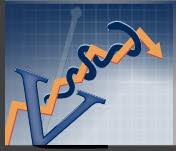
Dicks commentary bug
What is the difference between describing how and explaining why? To describe how means to reconstruct the series of specific events that led from one point to another. To explain why means to find causal connections that account for the occurrence of the particular series of events to the exclusion of all others.
— Yuval Noah Harari, in "Sapiens"
Studies and discussions about veterinary markets historically have focused on the how and the what. The why has been more elusive. Consider a meeting in 2016 at Michigan State University College of Veterinary Medicine co-hosted by the American Veterinary Medical Association and the Association of American Veterinary Medical Colleges, dubbed Fix the Debt Summit. The what clearly was identified as the rising debt-to-income ratio among new veterinary school graduates. The how was defined as the rising cost of education and slower growth in veterinary incomes.
The why, however, was presented as a set of hypotheses: Students borrow too much and spend money unwisely. Colleges have too much administration and are wasteful. Declines in public funding in education raised institutions' share of costs. New students are not ready to practice medicine. There is a general lack of financial literacy. Et cetera.
But no statistically valid analysis was presented on the relative importance of each of the hypothesized causes. We found this troubling. How can you solve a problem without knowing why it exists?
Introducing data analytics
Data analytics refers to the techniques and processes of collecting, inspecting, cleaning, transforming, and modeling descriptive (qualitative) and mathematical (quantitative) information with the purpose of understanding a problem and why it exists. In discovering the causes, the process lends itself to evidence-based problem solving. Data analytics is therefore the foundation for efficiently reaching goals and objectives.
Michael R. Dicks 230
Photo by Scott Nolan
Michael R. Dicks directed the American Veterinary Medical Association Veterinary Economics Division from 2013 to 2018.
Imagine 40 dogs in a dog park. You are asked to evaluate their health by observation. While you might be able to spot some problems through observation alone, these would be hypotheses until you investigated further.
The standard for evidence-based veterinary medicine is to take the patient’s history, perform a physical exam, apply the appropriate diagnostics for the hypothesized problem, arrive at a diagnosis based on evidence (data) from those diagnostics, develop a treatment plan using outcome-based research and finally, to check that the treatment has been effective.
This is data analytics in action.
Agriculture is one example of a sector that employs a robust data-analytics system. Data on the purchase of inputs (seed, feed, fertilizer, equipment, labor and so on), the consumption of products and services, and everything in between is collected by federal and state governments, farm and commodity associations, universities and others, in a cooperative effort. The data is used to help players make better decisions, and hence, we have one of the lowest cost, most varied, most abundant and safest food supplies in the world.
Agriculture isn't unique in using data analytics. Other sectors that incorporate it include banking and finance, insurance and food services.
Full disclosure: Last summer, we started a business, Erupt LLC, that offers data analytics and educational outreach services to clients in the veterinary profession. We hope no one misconstrues our intent: We're advocating data analytics here not to raise our business's profile. Data analytics long has been a go-to tool in economics. We believe in its power to define and solve problems. In any case, the scale of analytics we recommend for the veterinary markets is beyond the scope of our startup. And AVMA still has a well-staffed economics division fully capable of doing the needed industry analysis.
Not indispensable but much more efficient
Evidence for why a problem exists is not always required to find a working solution. Franklin Roosevelt's New Deal lasted a decade and encompassed a litany of programs and policies that were tried and discarded. Roosevelt claimed he would try a program or policy to help end the Depression, and if it worked, he would deploy it. If it failed, he would assess the failure and try something else.
Trial and error eventually works but it uses more resources than necessary and takes longer than attacking a problem with data analytics. Here's an example of one successful application of data analytics, from my (Dicks') time teaching at Oklahoma State University: In the late 1990s, under constant budget pressure due to declining state funding, several Midwest land grant universities identified low freshman retention rates as a potential source of income. A 1% increase in the retention rate would net more than $1 million in revenue. With retention rates in the low 70% range, a lot of money was being left on the table.
Dr. Melissa Maddux
Dr. Melissa Maddux, an AVMA Economics Fellow in 2016-2017, contributed to research and helped communicate economics concepts to a veterinary audience.
The common belief was that low retention was driven by lack of affordability. After all, when asked why they left, students most frequently said they couldn't afford school, and most had balances on their university accounts, which seemed to support the claim. However, several of us with backgrounds in studying behavior wondered whether students were giving the answer that they perceived as socially acceptable or expected.
To test this, we designed a study in which we identified factors to predict which freshmen would not return. For those who did return, we asked why they wanted to return. Two factors dominated their responses: 1) They had made a connection with at least one faculty member; and 2) They were involved in at least one extracurricular activity.
In response, the universities established programs to connect more freshmen to faculty and incentivize extracurricular engagement. Within three years, retention rates improved appreciably. Moreover, students in these programs were found to have higher average grades than nonparticipants. In this way, data analytics helped students and the universities alike.
Data analytics can do the same for veterinary medicine. Here's just one possible application:
The American Heartworm Society's current recommendation for heartworm prevention in the United States is year-round, without seasonal pauses. Yet the average dog receives fewer than three months' worth of heartworm preventative per year, according to the veterinary data company Animalytics.
With the cooperation of pharmaceutical manufacturers and distributors, this private data-analytics firm is able to determine the number of doses of core vaccines and heartworm preventative provided to every veterinary practice location, including mobile and vaccine clinics. (A total of 31,000 locations receive shipments from manufacturers.) The data provides a measure of the number of doses of heartworm medications per dog, giving us the what. To understand the why, we need answers to many more questions.
Are low rates of compliance caused by high prices, low perceived value or both? How do geographic location, income or occupation of pet owners impact compliance rates? Do pet owners understand the risk of heartworm to their pets? Do they understand what it would cost to treat a pet infected with heartworm?
Also, how effectively does following the recommendation minimize or eliminate heartworm? What is the relationship between the average number of months of parasiticide given to the pet and the number of heartworm cases diagnosed?
To obtain answers, we need data on pet owners associated with the number of doses they purchase. Pet-owner surveys could supply some insight; information from veterinarians on pet owners’ actual purchases of heartworm control products would fill out the picture. Understanding pet owners' decisions would enable veterinarians to either adjust the recommendations or try new ways to communicate them.
Another example: Tens of millions of dollars have been spent on Partners for Healthy Pets, an initiative of a coalition of professional associations, veterinary colleges and animal-health companies to improve access to care and compliance. A major component of this initiative is forward booking — that is, scheduling the patient's next appointment before the client leaves an existing appointment. This is easily done. So why isn't it a common practice?
A veterinary technician at a hospital where I (Dicks) was helping to implement the strategy might have put her finger on the explanation. She asked: "Is there any evidence that it will work?"
The question stunned me. I had to admit, "We know it works elsewhere but we don't know that it works in veterinary medicine. We don't have any data."
The profession should not use scarce resources on initiatives with no accountability any more than a veterinary practice should ask clients to purchase a service that has no demonstrated effect on improving pet health.
The profession's present relationship with data
The veterinary profession generally defaults to the trial-and-error method of problem-solving. As a result, problems go unresolved as newer, more immediate problems take hold. Because the time needed for the trial-and-error method to yield solutions vastly exceeds the longevity of each "most important" problem, evidence-based solutions are never identified or implemented.
Many professional associations and private organizations have collected and analyzed data intermittently to answer the what and how of various problems, but no one has continuously and consistently collected the comprehensive set of data needed to enable ongoing analysis of the veterinary markets. And much of the collected data is from surveys rather than from actual transactions or measurements of other actions. While both types of data when collected over time can indicate how an event is changing, they don't necessarily give equally reliable data about why something is happening. Responses to surveys are subjective, and respondents are apt to give answers that are inexact or that they perceive the survey taker expects to hear (a phenomenon known as social bias).
Some sectors of veterinary medicine do share data for analysis. For example, U.S-accredited colleges of veterinary medicine provide information to the Association of American Veterinary Medical Colleges. Members of groups such as the American Animal Hospital Association and Veterinary Management Groups collect and share practice information within their groups. Manufacturers and distributors enlist a single entity to aggregate sales data. Large practice chains aggregate the data of their hospitals to try to derive health-care and economic insights, most of which they keep private. Independent practice owners and other owners of practice groups likewise could pool their data. It could be anonymized for privacy and handled by an umbrella group that serves all contributors and participants equally.
What needs to be done?
Much data is being collected but it is owned by multiple separate collectors. They include product manufacturers, pet insurance companies, diagnostic laboratories, veterinary supply distributors, universities, professional associations, veterinary practices, practice information management system service providers and online pharmacies, to name a few.
For-profit companies amassing detailed practice data to promote products and services and sometimes to sell to others has raised concerns about data privacy and data ownership. There are few guidelines or rules about how practice data should be handled in the U.S., and the potential benefits of this information to the profession and animal health may get lost in the shuffle.
Why should private entities want to share their information, in an age when information is the coin of the realm? And why should practices entrust their valuable client data to companies that may treat the information as a commodity? In data-sharing, overcoming distrust is a big and legitimate challenge.
Another obstacle to deriving meaningful insights from practice data, in particular, is the data itself. Practice information management systems aren't designed for collecting data for analytics purposes, and the profession lacks a shared, standard language that would make comparisons among records doable. Further, there are no incentives or penalties to motivate veterinarians and industry to keep better records for research.
But these challenges are all worth taking on and working through because evidence-based solutions will support the overarching goal of improving the human-animal bond. The stronger the bond, the greater the willingness of pet owners to follow veterinary care recommendations, which improves the lives of pets, pet owners and those who provide and support animal health services.
And here's the thing: As the number of unresolved challenges grows ever larger, the incentive increases for companies with deep pockets and data know-how to develop their own approaches to collecting and parsing veterinary data for purposes that might not help sustain the veterinary profession.
For instance, it's well-known that veterinary students graduate with hefty educational debt. Companies with no particular commitment to the profession abound offering products and services to new veterinarians in the name of "debt relief." Whether they can provide genuine solutions to individuals is questionable. Certainly, they're not providing solutions to the profession's collective problem of high student debt.
Disparate entities invested in the veterinary profession must learn to collaborate in order to provide the comprehensive and consistent information needed to elucidate why specific problems exist. Only through cooperation and instituting sound data analytic processes can we learn to manage and minimize persistent problems.
In sum, the better the data, the better the analysis, the more efficient the problem-solving strategies, the more smoothly running the veterinary markets, the better the patient care and greater the professional satisfaction.
Next week: Choosing a path at a critical crossroads
About the authors:
Michael Dicks, PhD, established the veterinary economics division of the American Veterinary Medical Association in 2013 and was its director until 2018. Dicks earned a BS in biochemistry and animal science from California Polytechnic State University, taught chemistry as a Peace Corps volunteer in Kenya, then completed a master's and doctorate in agricultural economics at the University of Missouri.
He was employed by the U.S. Department of Agriculture Economic Research Service for five years before joining the faculty at Oklahoma State University, where he spent nearly 24 years prior to his stint at the AVMA. Today, Dicks works as a consultant in several capacities, including as "chief data human" at Erupt LLC, which provides data analytics and educational outreach to clients in the veterinary realm.
Melissa Maddux, DVM, is a 2016 graduate of the University of Tennessee College of Veterinary Medicine, a former AVMA economics fellow and CEO of Erupt. She practices equine and small animal medicine in Knoxville.